07.10.2024 by Anete Ezera
Data visualization, a powerful tool for data analysts, media professionals, businesses, and more, can transform raw data into meaningful, understandable visuals. It’s like translating a foreign language, making complex information accessible to everyone. By using different types of data visualizations such as charts, graphs, and maps, we can quickly identify trends, patterns, and outliers that might otherwise remain hidden.
You can use data visualization types like infographics to simplify complex ideas or charts to spot data trends. But how do you know what type of data visualization to select for your specific project? Or perhaps you’re wondering, what data visualization types are out there to choose from?
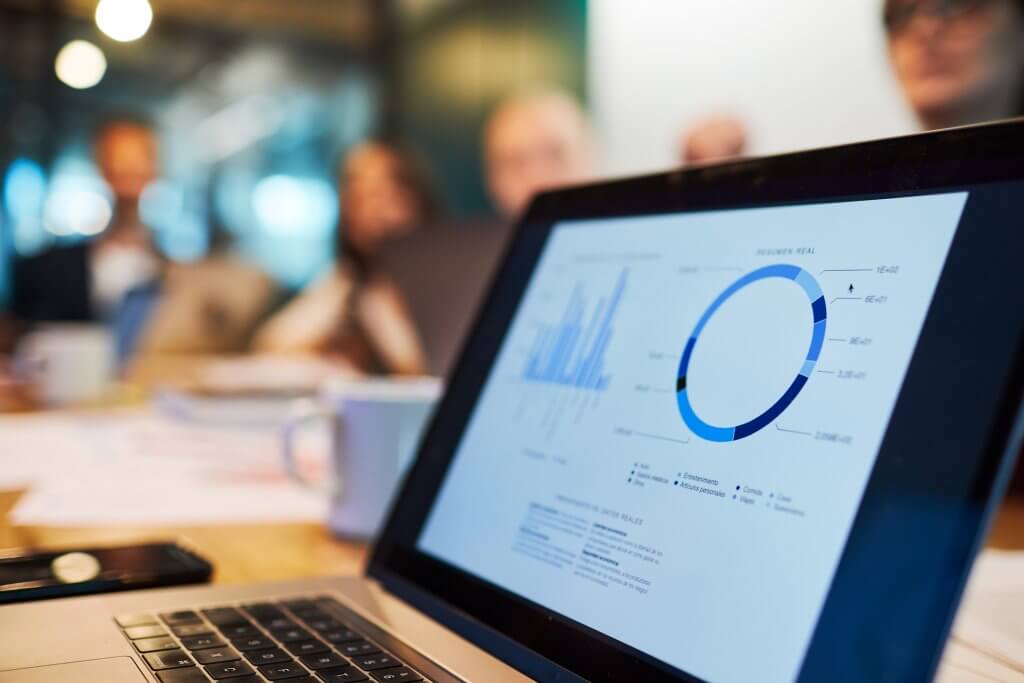
In this article, we’ll explore the various types of data visualizations available and discuss when and why to use each one. Also, we’ll explore different ways of categorizing data visualization types. So whether you’re a seasoned data analyst or just starting your journey into data, understanding these techniques will help you communicate your findings effectively and make informed decisions.
Lastly, we’ll discuss Infogram, an interactive AI data visualization tool that makes creating different types of data visualizations easy and quick. Let’s dive in and explore how you can leverage different data visualization types and create them!
The many ways of categorizing data visualization types
While there are many different types of data visualizations, there are also numerous ways to categorize them. So before we group and list different data visualization types, let’s explore the versatility of methods for categorizing data visualizations. Plus, establish how each method can provide unique value in understanding the wide array of data visualization types.
1. Purpose-based categorization
One of the most intuitive ways to categorize data visualizations is by examining their purpose. This method focuses on understanding why the visualization exists and what it aims to achieve. Purpose-based categorization reflects the intent behind the visualization and emphasizes how it fulfills specific communication, analysis, or storytelling needs.
For example, types of data visualizations may be exploratory, explanatory, monitoring, or communicative. When using this method, you’ll discover how each visualization helps answer questions, identify patterns, or communicate messages. However, this approach may not be granular enough to capture the full diversity of visualization types.
2. Attribute-based categorization
Another effective way to categorize types of data visualizations is through their attributes or characteristics. This examines the structural elements of visualizations, such as the data types they represent, the visual techniques they use, or the level of interactivity they offer.
For example, attribute-based categorization might differentiate data visualizations based on whether they handle categorical, numerical, or temporal data or by the visual elements they use, such as lines, bars, colors, or shapes. This method is deeply rooted in the actual components of the visualization itself, providing a detailed and technical understanding of each type. Nevertheless, categorizing data visualizations this way could be too overwhelming because of the complexity and number of attributes.
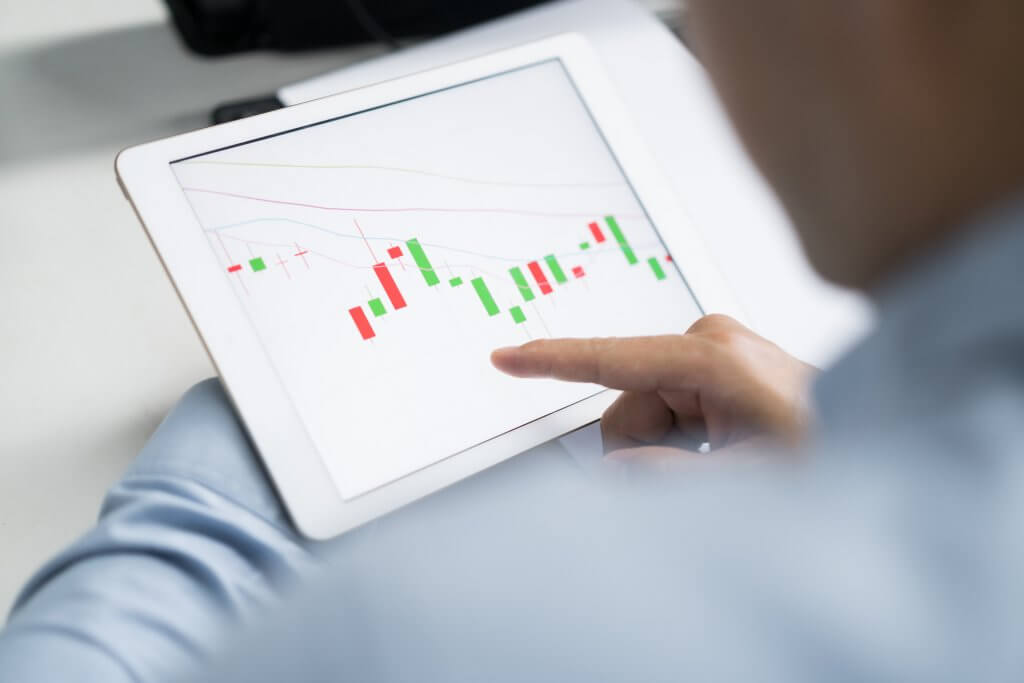
3. Format-based categorization
Format-based categorization groups data visualization types according to the medium or format in which they are presented. This can include static vs. interactive visualizations, animated vs. still images, or even web-based vs. print formats.
This method focuses on how the visualization is consumed and interacted with by the audience. For example, dashboards are often interactive and dynamic, allowing users to explore data, whereas reports are typically static and designed to present information in a fixed, consumable format.
4. Data complexity-based categorization
Visualizations can also be categorized by the complexity of the data they represent. This method focuses on whether a visualization handles simple or complex datasets, the number of variables involved, and the sophistication of the relationships depicted.
For example, simple visualizations like pie charts or bar charts represent basic, one-dimensional data, while complex visualizations such as network diagrams or multi-variable heatmaps handle intricate datasets with numerous dimensions and interactions.
5. Audience-based categorization
The audience for whom the data visualization type is intended can also serve as a basis for categorization. This method considers whether the visualization is designed for data experts, general audiences, business professionals, or academic researchers.
For instance, visualizations for expert audiences might include complex scatter plots or statistical diagrams, while general audiences might be better served by simpler, more intuitive visuals like pie charts or infographics.
6. Hybrid categorization
Many real-world visualizations don’t fit neatly into a single category. Hybrid categorization involves combining multiple methods to create a more comprehensive classification. For example, a dashboard might be categorized as interactive (format-based), explanatory (purpose-based), and designed for a business audience (audience-based).
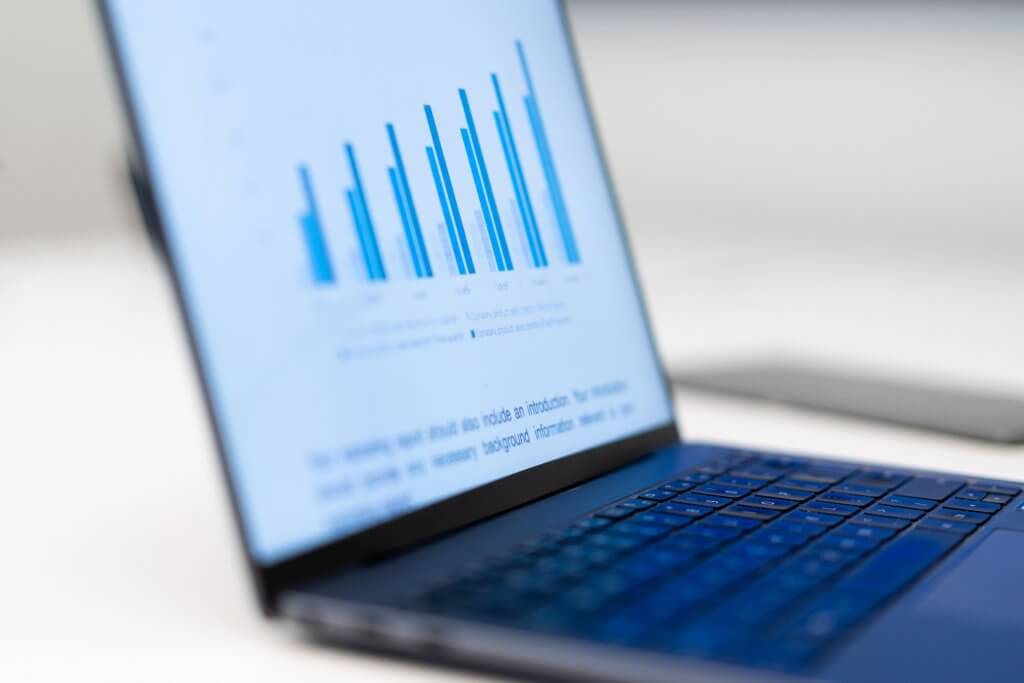
Hybrid categorization acknowledges the multidimensional nature of data visualizations and provides a richer, more nuanced understanding of their capabilities and applications.
Types of data visualizations
While there are many different ways you can group and categorize data visualizations, let’s list the following data visualization types by their purpose as this can offer the most context and insights on the visualizations’ main aim and value.
1. Compare
These visualizations are ideal for comparing different data points, categories, or illustrating distribution patterns over time or between groups. They help identify trends, patterns, and differences across various data sets.
Bar chart: Displays data with rectangular bars, either horizontally or vertically. Best for comparing discrete categories.
Column chart: Similar to bar charts but with vertical bars. Ideal for showing changes over time or comparing items across categories.
Line chart: Uses a line to connect data points over time, making it great for trend analysis.
Area charts (incl. stacked, stream, stepped): Similar to line charts but with the area below the line filled. Effective for showing cumulative trends or distributions over time.
Slope chart: Connects two points with lines to show changes between two time periods or categories, ideal for showing before-and-after comparisons.
Population pyramid charts: Two back-to-back bar charts representing different groups (e.g., age, gender) within a population, perfect for demographic data.
Candlestick charts: Commonly used in finance to show stock price movements, indicating opening, closing, high, and low prices over time.
Radar chart: Displays multivariate data in a radial form, useful for comparing multiple variables or performance across several metrics.
Best use cases
Comparison and distribution visualizations are particularly effective for tracking changes over time, making them ideal for time series analysis. For example, line charts and area charts are excellent choices when you need to observe trends, fluctuations, or patterns across different time periods. They work well for illustrating monthly sales trends, stock market performance, or website traffic over time.
When comparing different categories, bar charts and column charts excel at showing differences between groups or segments. These visualizations make it easy to compare items such as sales across different regions, survey responses by age group, or product popularity across different categories. Their straightforward format allows viewers to quickly grasp the differences between categories.
Best practices
To maximize the effectiveness of comparison and distribution charts, use consistent scales and intervals. This will help you easily interpret your data and accurately represent the differences between categories. For example, when comparing sales figures across months, ensure that the intervals on your axis are equally spaced to avoid misleading the viewer.
Also, avoid overloading the visualization with too many categories or lines. Instead, focus on the most relevant data points or categories, or consider breaking the data into smaller, more manageable charts if necessary.
Lastly, use contrasting colors to differentiate data points or categories clearly. This makes it easier for the audience to distinguish between different elements, improving the overall readability of the chart. However, be mindful of colorblind accessibility by using color palettes that are easy to differentiate for all viewers.
2. Composition and part-to-whole
These types of data visualizations highlight how individual parts contribute to a whole, showing proportions, percentages, or segments.
Pie charts (including doughnut, semi-circle, irregular): Circular charts divided into slices representing proportions of a whole. Doughnut charts are similar but have a hole in the center.
Stacked bar/column chart: Bars stacked on top of each other to show the breakdown of categories within a total.
Treemaps: Uses nested rectangles to display hierarchical data, with the size and color representing different variables.
Funnel charts: Visualizes a process or stages, often representing conversion rates, where the width of each stage indicates its value.
Best use cases
Composition visualizations are highly effective for showing how different parts contribute to a whole, making them particularly useful for representing proportions, compositions, or hierarchical data. For example, pie charts and stacked charts are ideal for displaying market share, budget allocations, or sales distribution among different categories.
Treemaps are excellent for visualizing hierarchical data or compositions, especially when you need to present complex datasets with multiple layers or categories. They can help identify which elements occupy the largest portion within a hierarchy, such as sales contributions by product category and sub-category or website traffic by source and sub-source.
Funnel charts are perfect for tracking drop-offs in processes or stages, such as sales conversion rates, lead generation funnels, or website navigation flows. They visually depict how data flows through a process, allowing you to pinpoint stages where significant drop-offs or losses occur, which can be essential for optimizing sales or marketing strategies.
Best practices
When using pie charts, limit them to simple comparisons with 2-6 segments. Beyond this range, pie charts can become cluttered and difficult to interpret, making it harder for viewers to distinguish between the segments. For more complex data, consider alternative visualizations like stacked bar charts or treemaps.
For stacked charts and treemaps, ensure that you use clear labels and consistent color coding to help viewers quickly identify and differentiate between categories. This practice improves readability and allows your audience to easily understand the composition.
When creating funnel charts, maintain a logical order that accurately represents the progression of stages in the process. This ensures that the visualization reflects a clear and understandable flow, helping viewers track how data moves through each stage.
3. Distribution and trends
These types of data visualizations illustrate patterns, distributions, and trends in data, showing how data points are spread across a range or how variables relate.
Scatter plots: Plots data points on an x and y-axis to show relationships or distributions. Great for correlation analysis.
Bubble charts: An extension of scatter plots where data points are represented as bubbles, with bubble size indicating an additional variable.
Area charts: Relevant for distribution when depicting cumulative data.
Best use cases
Distribution and trends visualizations are ideal for understanding how data points are spread across a range, identifying relationships between variables, or tracking cumulative values over time. Scatter plots are particularly useful for identifying relationships or correlations between two variables, making them a powerful tool for statistical analysis.
Bubble charts take this a step further by representing multi-dimensional data, where an additional variable is visualized through the size of the bubbles. This makes bubble charts suitable for complex data comparisons, such as analyzing the profitability, market share, and sales volume of different products simultaneously. They provide a more comprehensive view of how multiple factors relate to each other, making them great for business, marketing, or economic data analysis.
Area charts are best used for showing cumulative values over time, providing a visual representation of how different categories contribute to a total trend. For instance, they work well for displaying total revenue over time while also showing the contribution of individual product lines to the overall figure.
Best practices
When using scatter plots, include trend lines to highlight correlations or patterns between variables. Trend lines help viewers quickly identify whether there is a positive, negative, or no correlation, making the visualization more informative and easier to interpret.
For bubble charts, it’s important to limit the number of bubbles to avoid visual clutter. Too many bubbles can make the chart difficult to read and can overwhelm viewers, so focus on the most relevant data points or categories.
Lastly, always ensure that the scale accurately represents the data’s range in all distribution and trends charts. Inaccurate scaling can distort the data, leading to misleading interpretations. Using a consistent and accurate scale helps maintain the integrity of the visualization and ensures that the audience can draw correct conclusions from the data.
4. Relationships and flow
The following data visualization types best display the relationships, connections, or flow of data between different categories or groups, effectively showing movement or transition.
Sankey chart: Shows the flow and proportions between different stages or categories.
Alluvial chart: Similar to Sankey charts but often used for categorical data with changes over time.
Best use cases
Relationships and flow data visualizations types are designed to illustrate how data moves between different categories or stages, making them ideal for understanding the flow of resources, processes, or connections within a system. Sankey charts and alluvial charts are particularly effective for visualizing data movement between categories, allowing you to see how different elements connect, transition, or change over time.
Best practices
When creating relationship and flow visualizations, it’s crucial to use distinct colors to differentiate flows. This helps viewers easily distinguish between different paths, categories, or connections, making the chart more intuitive and easier to understand. Choose contrasting colors to ensure that each flow stands out clearly, and consider using a consistent color scheme if categories are related.
It’s also important to keep the number of categories reasonable to avoid confusion. Including too many categories or flows can result in a cluttered visualization, making it difficult for viewers to follow the movement of data. Focus on the most significant or relevant flows to maintain clarity and ensure that your visualization remains easy to interpret.
Finally, always label connections clearly to maintain readability. This includes labeling both the categories and the flow lines themselves so that viewers can quickly understand the relationships being presented.
5. Maps and geographic data
These types of data visualizations are ideal for representing spatial or geographic information.
Abstract map: General representation of spatial data.
Choropleth map: Uses color gradients to indicate different data values across geographic regions.
Icon map: Places icons on a map to represent different data points.
Best use cases
Maps and similar types of data visualizations are highly effective for displaying data such as population density, where you can visualize how populations are distributed across different areas, or for showing sales regions, helping businesses understand where their products are performing well or where there’s potential for growth. These data visualizations types are also excellent for representing weather patterns, such as temperature variations, rainfall distribution, or wind speeds across different geographic areas. By using maps, you can effectively illustrate patterns, trends, or relationships within your data that are tied to specific locations, providing valuable insights into spatial dynamics.
Best practices
When using map visualizations, it’s crucial to choose the appropriate map projection for your region or data type. Different map projections can distort the size or shape of areas, so selecting the right one ensures that your visualization accurately represents the geographic information.
Additionally, be mindful of the number of categories or colors used in your map. Avoid using too many categories or color gradients, as this can overwhelm viewers and make it difficult to distinguish between different data points. Instead, use a clear and simple color scheme, with contrasting shades to highlight the most important data. This improves readability and ensures that the audience can quickly identify patterns or trends in the geographic data.
6. Progress and project management visualizations
Progress and project management visualizations are designed to track timelines, milestones, progress, or stages within a project. These types of charts provide a clear overview of how a project is advancing, helping teams monitor progress, manage resources, and stay on schedule.
Gantt charts: Gantt charts display tasks, activities, or phases over time, showing start and end dates, task duration, and dependencies.
Progress bar charts: Simple and effective, progress bar charts use horizontal bars to represent the completion percentage of tasks, projects, or goals. They are especially useful for tracking the status of individual tasks or the overall completion of multiple projects in a concise format.
Best use cases
Gantt charts are ideal for outlining the stages of a project, identifying task dependencies, and monitoring progress, making them indispensable for managing complex projects with multiple stakeholders. Plus, both Gantt charts and progress bar charts are helpful for scheduling tasks, assigning resources, and ensuring that team members understand the timeline and priorities.
Best practices
For Gantt charts, always include clear time intervals (e.g., days, weeks, or months) to provide a clear sense of the timeline. Also, use color coding to distinguish different task categories, phases, or statuses, ensuring that viewers can quickly identify the status or priority of each task.
However, for progress bar charts, make sure to keep the design simple and focus on displaying progress percentages clearly, ensuring that the visualization remains easy to read and interpret.
7. Facts, figures, and summaries visualizations
Facts, figures, and summaries visualizations focus on emphasizing specific data points, statistics, or summarizations. These types of visualizations are designed to highlight essential information clearly and concisely, making them ideal for communicating critical insights at a glance.
Facts and figures boxes: These visual elements showcase key statistics or important numbers in a visually appealing format.
Tables: Tables provide a structured format for displaying detailed data, allowing for easy comparison and analysis.
Best use cases
Facts and figures boxes are excellent for quickly communicating vital statistics. However, tables are ideal for showcasing comprehensive data that requires careful scrutiny.
Best practices
Use these types of data visualizations sparingly to avoid overwhelming viewers with excessive text or information. Keeping the presentation concise helps maintain the audience’s focus on the most crucial data points. Also, ensure that numbers in facts and figures boxes are large and clear for quick interpretation. This allows viewers to grasp the information at a glance without straining their eyes.
8. Reporting and multi-dimensional analysis visualizations
Reporting and multi-dimensional analysis visualizations are designed to consolidate multiple data points or types into a single view, offering comprehensive insights. These visualizations enable users to analyze complex datasets from various angles. Furthermore, they empower users to make informed decisions based on a holistic understanding of the data.
Infographics: Infographics combine various visual elements, such as icons, charts, and text, to tell a cohesive data story. They effectively convey complex information in a visually engaging manner, making them suitable for educational purposes or marketing materials.
Reports: Reports present data in a detailed format, often incorporating multiple visualization types to provide an in-depth analysis. They are valuable for stakeholders needing a comprehensive overview of performance, trends, or findings.
Dashboards: Dashboards serve as interactive platforms that showcase various metrics and key performance indicators (KPIs) in real-time. They provide a snapshot of an organization’s performance, allowing users to monitor progress and make data-driven decisions quickly.
Best use cases
Infographics are ideal for summarizing intricate information in an engaging format, while reports offer detailed analyses for thorough examination. However, dashboards are invaluable for monitoring key metrics and enabling rapid responses to changing conditions or performance indicators.
Best practices
For these types of data visualizations it’s vital to maintain a consistent design and color scheme to create a cohesive look and feel. Also, pay attention to key metrics and insights to avoid cluttering the visualizations with unnecessary information. Prioritizing essential data helps viewers quickly understand the main takeaways without feeling overwhelmed.
Infogram: A tool for different types of data visualizations
Now that we’ve distinguished the different types of data visualizations, what tool should you choose to visualize them with? The answer is easy: Infogram.
Infogram is more than just a data visualization platform; it’s a creative toolkit designed to bring your data to life. With its intuitive interface and AI-driven features, Infogram empowers users of all skill levels to create stunning and interactive visuals that captivate and inform.
Key features of Infogram
Extensive chart library: Infogram offers a diverse range of chart types, from classic bar charts and line graphs to more specialized options like radar charts and treemaps. This flexibility ensures you can find the perfect visual representation for your data.
AI-powered suggestions: Infogram’s AI-driven recommendations provide valuable insights into the most effective chart types and design elements for your data. This intelligent guidance helps you create visualizations that are both visually appealing and informative.
Interactive elements: Infogram empowers you to enrich your visualizations with interactive elements such as tooltips, animations, and filters. These features make your visuals more engaging and allow your audience to explore the data in depth.
By leveraging the power of Infogram, you can:
- Create professional-quality visualizations: Infogram’s tools and features enable you to produce high-quality visuals that are visually stunning and easy to understand.
- Communicate complex ideas effectively: Infogram helps you transform complex data into clear and compelling narratives that resonate with your audience.
- Engage your audience: Infogram’s interactive elements and visually appealing designs capture attention and encourage exploration.
- Collaborate seamlessly with your team: Infogram’s real-time collaboration features facilitate efficient teamwork and knowledge sharing.
- Save time and effort: Infogram’s user-friendly interface and pre-designed templates streamline the visualization process, allowing you to focus on your data analysis.
All in all, Infogram is a powerful and versatile data visualization tool that empowers you to create stunning visuals that effectively communicate your insights and engage your audience. Whether you’re a data analyst or teacher, Infogram provides the tools and resources you need to succeed.
Different data visualization types: Final considerations
In conclusion, the diverse array of data visualization types serves as powerful tools for effectively communicating complex information and insights. Each visualization type, whether it be a bar chart, scatter plot, or dashboard, is tailored to address specific data needs and contexts, making it crucial to select the right one for the task at hand.
When choosing a data visualization type, consider the nature of the data, the story you wish to convey, and your audience’s needs. Different visualizations excel in different scenarios. For example, line charts are optimal for tracking trends over time, while maps are best suited for geographic data representation. By understanding the strengths and weaknesses of each type, you can ensure that your visualizations improve understanding.
And when it comes to choosing a data visualization tool, consider using Infogram, an interactive AI-powered tool. Infogram makes creating different data visualization types easier and quicker. With AI-powered functionalities like AI infographic generator and personalized AI chart suggester, you can create engaging and insightful data visualizations with ease. Sign up and try it out today!
Get data visualization tips every week:
New features, special offers, and exciting news about the world of data visualization.